Connect the dots with AIOps - Making intelligent DevOps work for you

Posted By
Shreya Nair
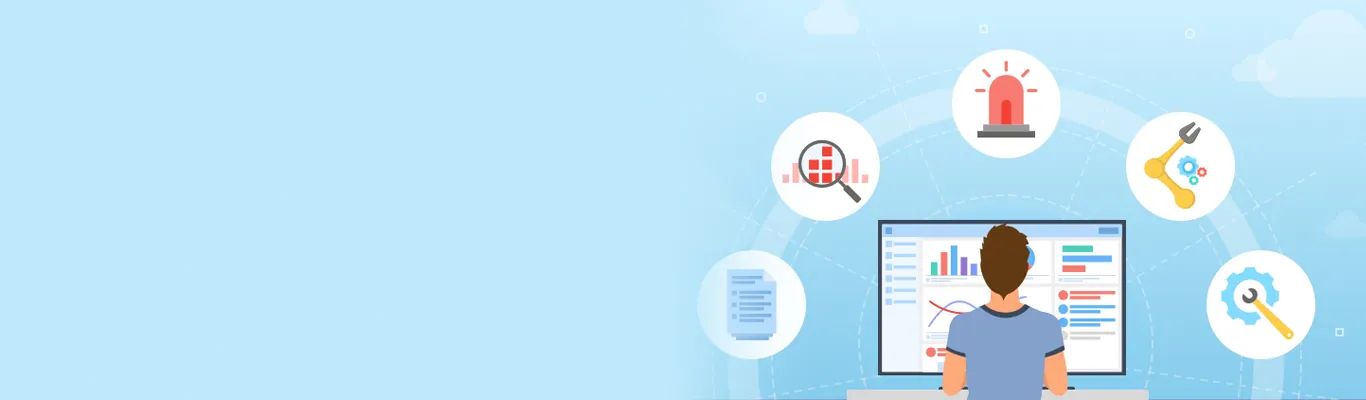
In our last pertinent blog, we discussed the futuristic AIOps and their myriad possibilities within the DevOps community. To recap, AIOps, or Algorithmic IT Ops, refers to solutions that use artificial intelligence and machine learning to automate tasks and processes, eventually reducing the required human intervention part to a minimum. This blog looks under the hood to explore the relevance of AIOps in the IT world today.
The need for AIOps arises from preemptively evading last-minute fires by self-learning predictive solutions based on machine-learning concepts. The new IT environments are becoming increasingly complex due to the popular adoption of IaaS, PaaS, and SaaS infrastructure powered by the omnipresent cloud technology. However, this also means that much time, effort, and resources are dedicated to monitoring and troubleshooting. This is a dangerously reactive position for any company to hold. AIOps advocates using change-tolerant algorithms to fix repetitive problems and using the vast volume of generated operational data to gain profitable insights into the business. This releases the teams from entrapment in mundane tasks and spends more time on proactive relevant tasks.
In general, organizations are recommended to build on the following three concepts:
- Decide the architecture strategy to be employed to accelerate DevOps integration.
- Develop a data strategy that drives digital business transformation.
- Develop a cognitive computing strategy based on advanced algorithms to maximize the business value of the accumulated data.
For any organization, a crucial element of migrating to DevOps and more Agile development practices involves taking advantage of application performance data running on the live infrastructure. Furthermore, an increasing number of business-critical applications are scaled and automated thusly, there is also a stark escalation in the precedented amount of metric data available to the developer.
The huge volume of information generated with a DevOps integration and historical data about the environment are key learning data sets for the AIOps Platform. These platforms use machine learning to understand and identify the system's normal behavior over time and identify red flags on any errant system behavior.
A typical AIOps platform:
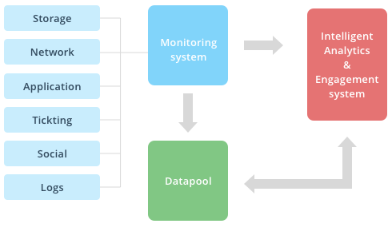
Monitoring system:
Proactive monitoring systems keep track of a number of relevant metrics. The criteria for monitoring success is based on the following:
- Identification and collection of key performance indicators.
- Machine learning technology and proactive anomaly detection.
- A consolidated monitoring view that includes performance and exception data.
Intelligent Analytics and Engagement System:
The Analytics component of the AIOps utilizes predictive algorithms, automated solutions, and alert mechanisms. As such, the component runs automated scripts for solutions and alerts concerned personnel or system with help from machine learning and artificial intelligence.
Pattern discovery and Anomaly detection:
One way for the early detection of any problems is by identifying irregular behavior based on consistent data monitoring. Instead of being penalized for a malfunction later on, early detection of potential issues aids in timely intervention before it escalates into a crisis. At its core, the premise for this argument is that it is possible to identify and separate normal system behavior from irregular behavior objectively. However, as in the case of any classification problem, there is a margin for error- for example, a normal behavior may be flagged as irregular, or an irregular behavior may pass undetected. This is the part where human interaction becomes inevitable. However, once a problem is handled by a human, the learning algorithms are quickly able to reevaluate and add the interaction to their learning set. Consequently, when a similar problem occurs again, the algorithm already knows how to behave based on the history of interactions.
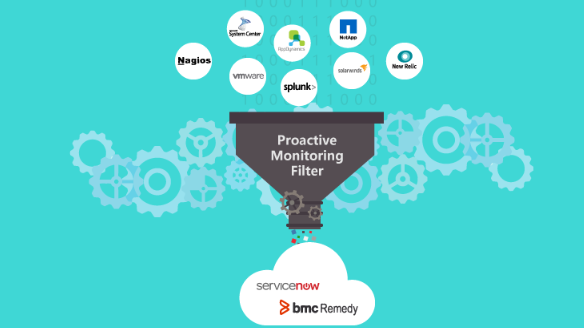
Predictive systems:
Predictive solutions are an integral section of the AIOps architecture. The process involves data collection, analysis, statistical analysis, and predictive modeling. This predictive model is deployed and monitored for precision and accuracy.
Data Pool:
The data pool maintains all the historical and real-time records, which will be analyzed with the help of algorithms, all tickets raised, all solutions suggested, and all the actions taken along with the results.
A closer look at AI in Ops
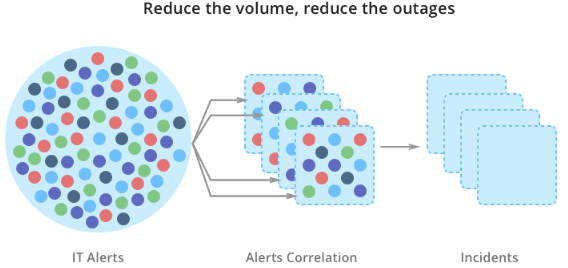
AIOps in action:
With the emergence of AIOps, it is easy to assume that ITOA (IT Operations Analytics) will go the way of the dinosaurs, however, it could not be further from the truth. Rather ITOA pertains to services around analyzing operational data from various sources, such as monitoring metric data, logs, security logs, etc.
AIOps works in tandem with ITOA to analyze the data generated by ITOA telemetry and understand the working of the IT environment. Subsequently, it can predict potential system failures, bugs, threats, and other issues while suggesting modifications to the existing environment to improve health, performance, and other metrics.
Currently, the marketplace offers many robust ITOA and AIOps products like Elastic, Hewlett Packard Enterprise, IBM, Splunk, and Sumo Logic, among many others.
The Machine learning perspective:
AIOps boasts a large arsenal of machine learning algorithms at its behest, which popularly includes association learning, clustering, recommendation engines, classification, similarity matching (as well as anomaly detection), neural networks, bayesian networks, and genetic algorithms. These algorithms are capable of solving complex business problems as described below:
- On the business operations side, AIOps analyze the IT and business data to identify behavior patterns that generate profitable business outcomes. Consequently, it can also identify new business opportunities and potential revenue streams based on the earlier behavior analysis.AIOps can also provide data-driven recommendations based on real-time and historical data to inform decision-making.
- Furthermore, pattern matching and anomaly detection can be supported by the AIOps platform with the help of robust fuzzy matching, neural nets, and clustering algorithms. A simple use case scenario would be to group multiple messages related to one fault or programming bug. For example, a programming error that creates database performance issues could result in hundreds of separate messages that humans would otherwise have to parse. Machine learning makes it easier to aggregate these collections of messages so that operations teams and developers can focus on the root cause of a problem.
- On a different note, with the rise of the cloud, there has been a shift from SNMP toward unstructured messages. Natural Language Processing techniques make sense of the messages like a human would. This adds the ability to read the context of a particular system/human interaction to the AIOps platform.
- Improvements in machine learning and integration with bug-tracking and issue-tracking services like JIRA and ServiceNow can help streamline performance-related bug-resolution processes.
In conclusion:
The colossal volume of application deployments daily, facilitated by the DevOps and automation culture, indicates evolving and complicated times for IT operations. The rapid advances in application development necessitate the need to handle increasingly complex system infrastructures while keeping track of performance, Mean-time-to-recovery, and change volume. This may well be out of bounds for handling by human capabilities soon. AIOps, replete with unsupervised and reinforcement learning algorithms, provide a special modus operandi to address this situation. It ensures that the human-machine interaction is more meaningful and fruitful by focusing on delivering key insights and reducing workloads.
In the face of such enticements, AIOps is definitely here to stay and more! To know more about AIOps, drop us a line
Related Blogs
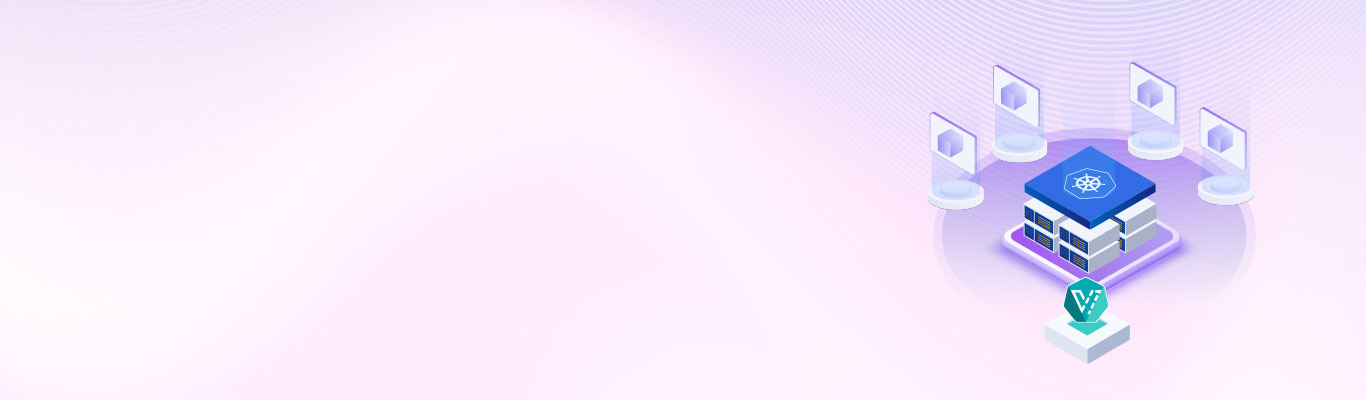
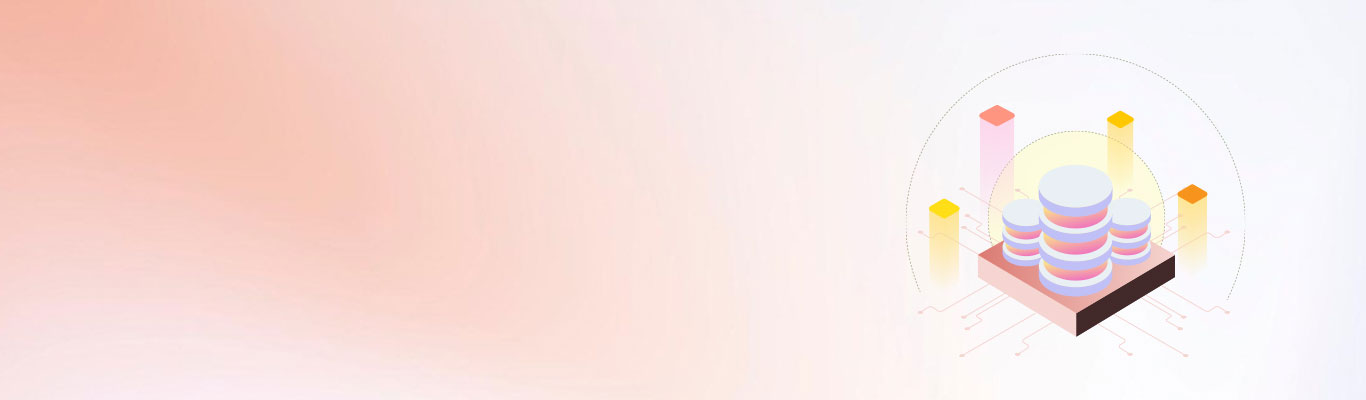